From GPT-1 to GPT-4: The AI Language Revolution
- Posted by Shruti Verma
- Categories Blog, College, Corporate, Individual, Trainers
- Date September 19, 2024
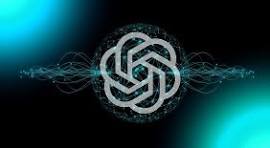
Introduction
In the realm of Natural Language Processing (NLP), Large Language Models (LLMs) have emerged as a cornerstone of artificial intelligence, revolutionizing our ability to interact with machines in a natural and intuitive manner. The Generative Pre-trained Transformer (GPT) series, developed by OpenAI, has played a pivotal role in driving the evolution of LLMs. This article explores the journey from the early days of GPT to the groundbreaking advancements achieved with GPT-4, and delves into the challenges and future prospects of this transformative technology.
Pre-GPT Era: Early Language Models
Before the advent of GPT, language models were primarily based on n-gram models and recurrent neural networks (RNNs). These models, while effective for certain tasks, struggled with capturing long-range dependencies and understanding context. Recurrent Neural Networks (RNNs), including Long Short-Term Memory (LSTM) variants, addressed some of these limitations but were still constrained by their sequential processing nature.
The Birth of GPT: GPT-1 (2018)
GPT-1, introduced by OpenAI in 2018, marked a significant breakthrough in LLM development. It leveraged the Transformer architecture, a novel neural network architecture that introduced the concept of self-attention mechanisms. This innovation allowed GPT-1 to process input sequences in parallel, enabling it to capture long-range dependencies and understand context more effectively than previous models.
Moreover, GPT-1 pioneered the approach of pre-training a language model on a massive dataset of text and then fine-tuning it for specific tasks. This unsupervised pre-training phase allowed GPT-1 to learn general language patterns and representations, making it more versatile and adaptable to various downstream applications.
GPT-2 (2019): Scaling Up
GPT-2, released in 2019, represented a substantial advancement over its predecessor. With a significantly larger model size and trained on a more extensive dataset, GPT-2 demonstrated remarkable capabilities in generating coherent and human-like text across a wide range of topics.
However, the release of GPT-2 was met with both excitement and concern. Its ability to generate high-quality, realistic text raised questions about potential misuse, leading OpenAI to initially withhold the full model. This decision sparked a debate about the ethical implications of powerful language models and the need for responsible development.
GPT-3 (2020): Unprecedented Scale
GPT-3, introduced in 2020, marked a quantum leap in LLM development. With an astonishing 175 billion parameters, it was the largest language model ever created at the time. This unprecedented scale enabled GPT-3 to achieve remarkable performance on a wide range of NLP tasks, including text generation, translation, question answering, and summarization.
One of the most notable features of GPT-3 was its ability to perform few-shot, one-shot, and even zero-shot learning. This meant that the model could adapt to new tasks with minimal or no additional training data, demonstrating its versatility and adaptability.
However, the sheer size of GPT-3 also raised concerns about its environmental impact. Training such a massive model requires significant computational resources, leading to a substantial carbon footprint. Addressing these environmental concerns is a critical challenge for future LLM development.
GPT-4 (2023): Enhanced Abilities
GPT-4, the latest iteration of the GPT series, represents a significant advancement over its predecessors. While specific details about its architecture and training data remain undisclosed, OpenAI has highlighted several key improvements:
Increased Reasoning Abilities:
GPT-4 has demonstrated enhanced reasoning skills, enabling it to perform complex tasks that require logical thinking and problem-solving.Improved Multilingual Capabilities:
GPT-4 has shown improved proficiency in multiple languages, making it more accessible to a global audience.Multimodal Capabilities:
GPT-4 can process and generate text, images, and other forms of media, opening up new possibilities for applications in areas such as design and content creation.
Challenges in the Evolution of GPT Models
As LLMs continue to evolve, several challenges persist:
Scalability vs. Efficiency:
Balancing the need for larger models with computational efficiency and cost is a constant challenge.Data and Privacy Concerns:
Accessing and managing vast amounts of data is essential for training LLMs, but it raises privacy concerns.Bias and Fairness:
Ensuring that LLMs are unbiased and fair is a critical ethical consideration.Environmental Impact:
The carbon footprint of training large-scale models is a growing concern.
Beyond GPT-4: The Future of LLMs
The future of LLMs is filled with exciting possibilities. As research progresses, we can expect to see even more powerful and versatile models that can understand and generate human language at an unprecedented level.
Key areas of future development include:
Enhanced Reasoning and Common Sense:
Developing LLMs that can reason about the world in a more human-like manner and apply common sense understanding.Domain-Specific Expertise:
Training LLMs to become experts in specific domains, such as healthcare, law, or science.Multimodal Capabilities:
Integrating LLMs with other modalities, such as images, audio, and video, to create more interactive and informative experiences.Ethical AI Development:
Ensuring that LLMs are developed and used responsibly, addressing concerns about bias, fairness, and privacy.
As LLMs continue to evolve, they have the potential to revolutionize various aspects of society, from education and healthcare to customer service and creative industries. However, it is essential to approach their development and deployment with a focus on ethical considerations and responsible AI practices.
About the Author: Shruti Verma is Skill Advisor at IDI Institute de Informatica. Learning for career is an Initiative of IDI that conducts courses in futuristic technologies with an aim to build SMART professionals where SMART is being Skilled, Motivated, Analytical, Resourceful and Transform people.
https://www.facebook.com/learningforcareer01
.
You may also like
AI Career Explosion: 50 Top Jobs Awaiting You
In the ever-evolving world of technology, Generative AI stands out as one of the most groundbreaking advancements.
Edge Computing: A Revolution at the Network’s Frontier
In the ever-evolving world of technology, Generative AI stands out as one of the most groundbreaking advancements.
Large Language Models: The Powerhouses of Modern AI
In the rapidly advancing world of artificial intelligence, Large Language Models (LLMs) have emerged as one of the most significant and transformative technologies. These models are not only pushing the boundaries of what AI can do but are also redefining our interactions with machines, from chatbots and virtual assistants to content creation and beyond.